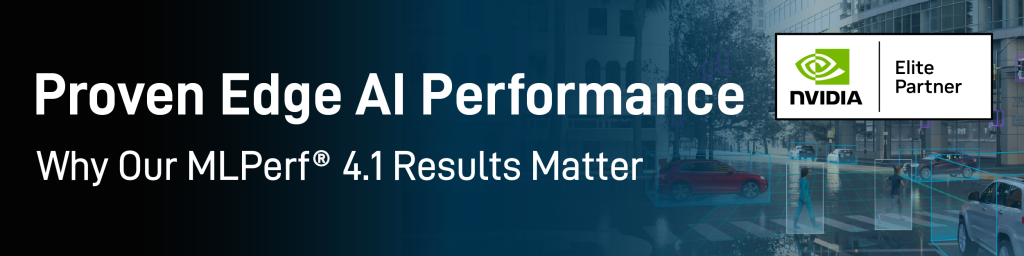
As AI continues to evolve, large language models (LLMs) have emerged as powerful tools for generating human-like text, processing natural language, and solving complex problems across a variety of industries. Among these LLMs, GPT-J 6B has become a notable tool for its ability to handle tasks such as text summarization, question answering, and content generation, making it a valuable asset in edge AI deployments.
Running LLMs like GPT-J on edge devices—especially those optimized for performance, like the Anvil Embedded System paired with the NVIDIA® Jetson AGX Orin™ 64GB system-on-module (SOM) —unlocks significant potential for applications where real-time processing and minimal latency are critical. Our recent submission to the MLPerf® Inference v4.1: Edge benchmark puts the Anvil Embedded System through its paces in real-world AI tasks, including natural language processing and inference workloads, demonstrating its effectiveness and reliability.
Why These Results Matter for Edge AI
The MLPerf v4.1 Inference benchmark provides an in-depth look into the performance of edge AI devices, measuring key metrics such as Single Stream Latency (ms) and Offline Throughput (Samples/s). These metrics are crucial in evaluating how well devices like the Anvil Embedded System with Jetson AGX Orin 64GB SOM handle demanding AI workloads. Here’s a breakdown of the results and their implications:
- Single Stream Latency: The Anvil Embedded System achieved a single stream latency of 4,145.57 ms, which indicates how fast it processes individual input tasks, such as a query to an LLM like GPT-J. The Anvil Embedded System is designed with optimal thermal management, power efficiency, and interface flexibility for faster processing under industrial conditions. This makes a significant difference in applications like autonomous machines or robotic systems, where even a small reduction in latency can enhance decision-making and operational safety.
- Offline Throughput: The Anvil Embedded System recorded an offline throughput of 64.01 samples per second. The Anvil Embedded System’s rugged design also provides benefits for throughput in industrial environments. This design provides the high throughput needed in harsher production environments over extended periods of time. This advantage is particularly relevant in robotics, industrial automation, and smart cities, where batch processing of data such as multiple video streams or sensor data needs to be processed quickly and reliably, even in demanding conditions.
These results underscore the Anvil Embedded System’s ability to handle LLM workloads in real-world scenarios effectively. When processing tasks such as text summarization or natural language queries on GPT-J, the system can deliver the necessary performance without sacrificing robustness. Single stream latency is critical for real-time applications, such as smart robotics or natural language interfaces in autonomous machines, where rapid responses are vital for performance and safety. Offline throughput, on the other hand, is essential in environments requiring high-efficiency batch processing, such as predictive maintenance in factories or real-time monitoring in smart cities.
Generative AI Use Cases and LLMs at the Edge
Generative AI is rapidly transforming industries, enabling advanced capabilities at the edge where real-time decision-making is essential. With platforms built for edge AI and robotics like NVIDIA Jetson, powerful AI models, including LLMs, vision language models (VLMs), and other types of sophisticated AI models, can be deployed directly at the edge, offering practical solutions to real-world challenges without relying on constant cloud connectivity. By leveraging these generative AI applications, businesses can deploy advanced AI models directly at the edge for use cases such as:
- Smart Agriculture and Precision Farming: Generative AI can optimize agricultural practices. LLMs can process and summarize large datasets with information such as weather patterns, soil conditions, and historical crop yields, helping farmers make data-driven decisions in real time. VLMs deployed on edge devices like the Anvil Embedded System can monitor plant health, detect diseases or pests, and predict optimal harvest times by analyzing images and sensor data from fields. Other types of generative AI applications can also assist in voice-based interaction with agricultural machinery, making complex operations simpler.

- Stock Management and Inventory Control: LLMs can process textual data like supply chain information or sales reports to optimize stock levels and inventory management. Connect Tech’s Edge Devices can host these models to provide real-time inventory tracking and analysis directly on-site. VLMs can be used to scan product shelves or warehouse environments to help ensure stock accuracy, while the populated scenarios can also enable voice-controlled systems for seamless interaction with inventory management tools.
- Smart Cities and Infrastructure Monitoring: LLMs can be used to analyze vast textual datasets related to urban infrastructure, such as maintenance records, inspection reports, and energy consumption patterns. This data can be summarized into actionable insights for city planners and engineers. In such use cases, VLMs deployed on edge devices like the Anvil Embedded System, can monitor city infrastructure through visual inspections of bridges, roads, and other critical assets, detecting structural damage or signs of wear.
- Patient Monitoring and Elderly Care: In healthcare, LLMs deployed at the edge can analyze patient records and symptom descriptions to assist with diagnostics or monitor ongoing treatments, offering real-time summaries and recommendations for medical staff. On the other hand, VLMs can be used to help can monitor the conditions of patients, detecting changes that may signal a health concern. Other generative AI applications based on voice data can be used to create personal assistant systems for elderly patients, allowing them to request assistance or access health updates effortlessly, enhancing care in remote or under-resourced areas.
- Multilingual Communication in Diverse Settings: Some types of LLMs deployed at the edge can translate documents and conversational text in real-time, facilitating communication between people speaking different languages. This can be particularly beneficial in multinational organizations, or during humanitarian efforts in remote regions. LLMs can also enhance this capability by enabling voice-based translation, making interactions smoother in environments with language barriers.
- Industrial Automation and Predictive Maintenance: In industrial settings, LLMs can analyze machine logs, maintenance reports, and operational data to predict equipment failures and optimize maintenance schedules, reducing downtime. Vision-based language models can visually inspect equipment for wear or malfunction. Connect Tech’s Edge Devices bring these capabilities closer to the site, enhancing efficiency and reducing reliance on cloud infrastructure.

- Accident Reporting and Traffic Management: Advanced language models like LLMs can analyze reports from various sensors and human inputs to provide automated traffic incident summaries and actionable insights for authorities. VLMs running on devices such as the Anvil Embedded System can analyze real-time video feeds to detect accidents, traffic violations, or road obstructions in smart cities.
Generative AI—spanning LLMs, VLMs, and other types of generative AI models—holds immense potential for solving real-world challenges across various sectors. Devices like the Anvil Embedded System paired with the Jetson AGX Orin SOM are key enablers of this transformation, helping make AI capabilities more accessible, efficient, and responsive in environments where cloud connectivity may be limited or impractical.
Why These Results Matter for Connect Tech
At Connect Tech, joining MLPerf and submitting these benchmarks reflect our commitment to providing high-performance, industrial-grade AI solutions to our customers. As a member of MLCommons, we aim to showcase how our systems not only meet but exceed industry standards. Our participation in MLPerf is a way to demonstrate the true performance of production-ready edge AI hardware. While developer kits and commercial grade hardware act as excellent initial development and benchmarking systems, Connect Tech products, such as the Anvil Embedded System, are optimized not just for performance but for rugged, real-world environments.
Our MLPerf submission is more than a technical benchmark; it’s a clear signal to our customers that we can deliver high performance at the edge, even in the most demanding conditions. We know our customers care about reliability, especially when deploying AI in autonomous systems, smart manufacturing, and other mission-critical applications. For them, these results mean the difference between systems that simply perform well in a lab setting and those that maintain high performance in challenging industrial environments where heat, dust, power constraints, and other factors are in effect.
Benchmark submissions like MLPerf also help our customers make informed decisions when choosing hardware for their edge AI applications. Knowing that the Anvil system offers powerful performance with lower latency and robust throughput help assure them that they can depend on our solutions for real-time processing, high throughput analytics, and data-driven decision-making at the edge—without sacrificing durability or reliability.
Ultimately, Connect Tech’s participation in MLPerf is about reinforcing the trust our customers place in our solutions. We aim to provide verified performance metrics that show our platforms are not only competitive but also built to excel in industrial applications.

Connect Tech Edge Devices’ Capabilities in Industrial Environments
Connect Tech Edge Devices are built to perform in challenging industrial environments. With its rugged design, compact form factor, and advanced heat dissipation capabilities, the Anvil Embedded System can function effectively in rough conditions, including extreme temperatures, vibration, and dust-heavy environments. This makes it ideal for deploying AI in industries such as energy, manufacturing, robotics, logistics, transportation, and agriculture, where reliability and durability are as critical as performance.
These rugged capabilities mean that LLMs and VLMs can be deployed at the edge in remote locations or under extreme environmental constraints, allowing companies to make real-time decisions without depending on external cloud-based infrastructure.